Time Series Econometrics: Detecting Bubbles, Non-Linear Models, and Statistical Inference
Introduction
Time series econometrics is a crucial area of study that focuses on analyzing data collected over time. This field is particularly important for understanding economic trends, forecasting future events, and detecting market anomalies like bubbles and crashes. This blog delves into the econometric detection of bubbles and crashes, specification testing and forecasting in non-linear time-series models, and the role of theoretical econometrics and statistical inference. These topics are vital for economists, policymakers, and financial analysts who rely on time series data to make informed decisions.
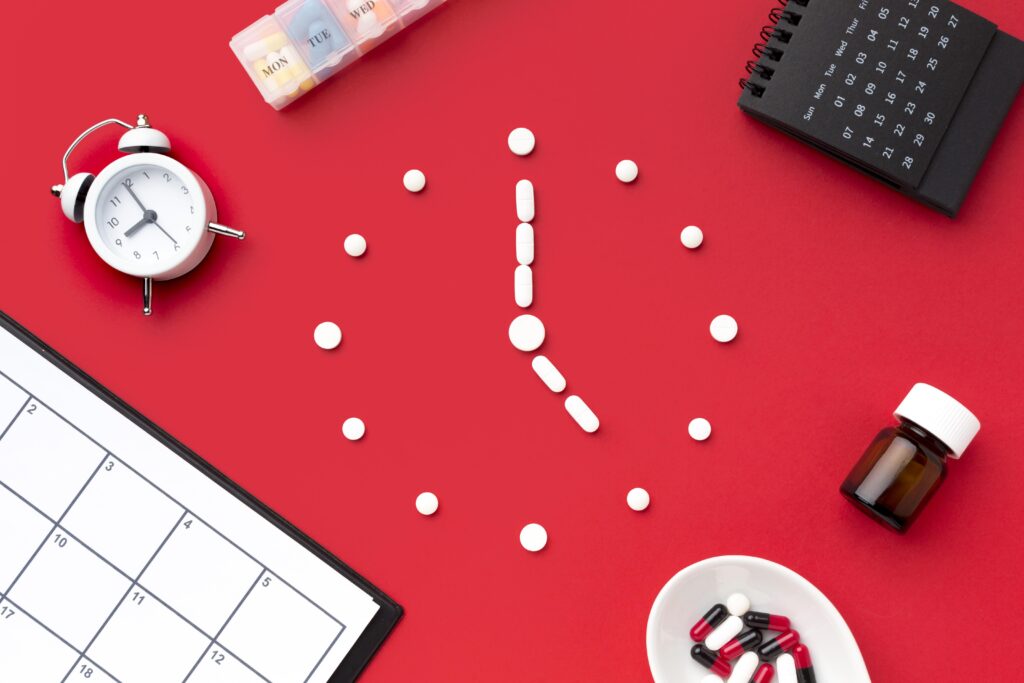
Econometric Detection of Bubbles and Crashes
One of the most significant applications of time series econometrics is the detection of financial bubbles and crashes. Bubbles occur when asset prices rise rapidly and exceed their intrinsic value, often followed by a sharp decline (a crash). Identifying these bubbles before they burst is crucial for preventing economic crises. Econometric techniques, such as unit root tests, cointegration analysis, and explosive root tests, are commonly used to detect bubbles in financial markets.
For example, the Generalized Supremum Augmented Dickey-Fuller (GSADF) test is a popular method for detecting bubbles. It allows for the identification of multiple bubbles within a time series by testing for explosive behavior in asset prices over time. Detecting bubbles early can help investors, regulators, and policymakers take preemptive action to mitigate the impact of a potential crash.
Specification Testing and Forecasting in Non-Linear Econometric/Time-Series Models
Time series data often exhibit non-linear patterns that cannot be captured by traditional linear models. Non-linear models, such as Threshold Autoregressive (TAR), Smooth Transition Autoregressive (STAR), and Generalized Autoregressive Conditional Heteroskedasticity (GARCH) models, are essential for capturing the complexities of economic time series. Specification testing in non-linear models ensures that the chosen model accurately reflects the underlying data-generating process.
Forecasting using non-linear models can provide more accurate predictions in the presence of structural breaks, regime changes, or other non-linear dynamics. For instance, GARCH models are widely used in financial econometrics to model and forecast volatility, which is inherently non-linear and time-varying. Accurate forecasting is crucial for risk management, investment strategies, and policy formulation.
Theoretical Econometrics and Statistical Inference
Theoretical econometrics provides the foundation for developing and testing econometric models. It involves the formulation of hypotheses, derivation of estimators, and evaluation of their properties, such as consistency, efficiency, and asymptotic distribution. Statistical inference, on the other hand, involves drawing conclusions about the population based on sample data.
In time series econometrics, statistical inference plays a key role in hypothesis testing, confidence interval estimation, and model validation. Techniques like Maximum Likelihood Estimation (MLE) and Generalized Method of Moments (GMM) are commonly used for parameter estimation in time series models. These methods allow economists to make reliable inferences about economic relationships and to validate models against observed data.
Conclusion: The Power of Time Series Econometrics
Time series econometrics is a powerful tool for understanding and predicting economic phenomena. By mastering the detection of bubbles and crashes, leveraging non-linear models for accurate forecasting, and applying rigorous statistical inference, economists and analysts can navigate the complexities of economic data with confidence. As financial markets and economic systems become more interconnected and volatile, the importance of time series econometrics will only continue to grow.
References
- Phillips, P. C. B., Shi, S., & Yu, J. (2015). “Testing for Multiple Bubbles: Historical Episodes of Exuberance and Collapse in the S&P 500.” International Economic Review, 56(4), 1043-1078. Available at: Testing for Multiple Bubbles
- Tsay, R. S. (1989). “Testing and Modelling Threshold Autoregressive Processes.” Journal of the American Statistical Association, 84(405), 231-240. Available at: Threshold Autoregressive Processes
- Bollerslev, T. (1986). “Generalized Autoregressive Conditional Heteroskedasticity.” Journal of Econometrics, 31(3), 307-327. Available at: GARCH Model
- Hamilton, J. D. (1994). “Time Series Analysis.” Princeton University Press. Available at: Time Series Analysis
- Hansen, L. P. (1982). “Large Sample Properties of Generalized Method of Moments Estimators.” Econometrica, 50(4), 1029-1054. Available at: GMM Estimators